5 Best Machine Learning APIs for Data Science
Machine Learning APIs make it easy for developers to develop predictive applications. Here we review 5 important Machine Learning APIs: IBM Watson, Microsoft Azure Machine Learning, Google Prediction API, Amazon Machine Learning API, and BigML.
Big data is streaming into businesses all over the Internet from various data sources like sensors, social media data, excel spreadsheets, reviews, customer data, etc. There are many companies like Google, IBM, Amazon, and Microsoft helping businesses process big data by building Machine Learning APIs so that organizations can make the best use of the machine learning technology.
Machine Learning is the big frontier in big data innovation but it is daunting for people who are not tech geeks or data science domain experts.Similar to how standard APIs help developers create applications, Machine Learning APIs make machine learning easy to use, for everyone. Machine Learning APIs abstract the complexities involved in creating and deploying machine learning models so that developers can focus on data munging, user experience, design, experimenting and delivering insights from data.
In the early days, machine learning algorithms and technologies were mostly used by scientists, tech geeks or domain experts. However, several organizations are now using Machine Learning APIs to make these technologies available to the masses. Machine Learning APIs make it easy for developers to apply machine learning to a dataset so as to add predictive features to their applications. Machine Learning APIs provide an abstraction layer for developers to integrate machine learning in real-world applications without having to worry about scaling the algorithms on their infrastructure and getting into the details of the machine learning algorithms. Machine Learning APIs require developers to focus on the two important aspects-
- Developers can concentrate on querying predictions by integrating Machine Learning APIs into their applications.
- Developers can track events on their applications to collect usage data.
Machine Learning APIs provide businesses with the ability to bring together predictive analytics so that they can get to know their customers better, understand their requirements and deliver products or services based on the past data trends, thereby initiating the selling process.There is an increasing percentage of real-time consumer interactions through Machine Learning APIs – making them an ideal option for exposing real-time predictive analytics to app developers.
Application developers always look for various ways to ease the lives of their users by introducing novel and innovative features that can help users save time. This is the reason for the popularity of Machine Learning APIs in app developers. Some standard examples of these APIs include Smart Tagging, Product Recommendations, Priority Filtering, and Spam Filtering.
Challenges Associated with using Machine Learning APIs

The most time consuming and important part of using machine learning APIs is identifying the business problem that needs to be solved and building meaningful datasets. This implies-
- Machine learning practitioners have to ensure that they are clear on what they need to predict or classify. Every problem cannot be solved with machine learning APIs so it is important to decide on whether the scenario fits or not.
- Machine learning practitioners should gather as much data as they can around a given context devoid of making several assumptions. This is important because data scientists or analysts will frequently find out unpredictable correlations between the input data and the column that they are trying to predict or classify i.e. the target value
Top Machine Learning APIs for Machine Learning Practitioners
The latest trend in Machine learning to be commoditized into a service which will develop into the mainstream as commoditized visualization and storage. Here are some of the best machine learning APIs that provide commoditized machine learning as a service (MLaaS) to business analysts and developers for application integration-
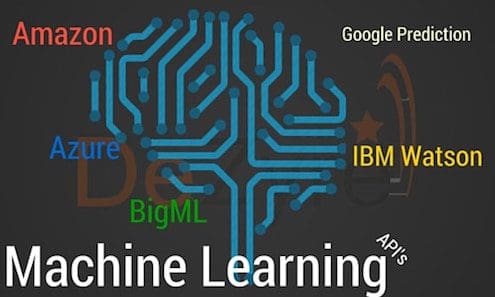
1) IBM Watson
For Machine Learning practitioners who are crunching at the moment to use IBM Watson’s machine intelligence service, within their mobile or web applications, need to gnaw no longer. IBM Watson API is a cognitive service that simplifies the process of preparing data and makes it easier to run a predictive analysis. It also provides the use of visual storytelling tools such as infographics, maps or graphs to exemplify analysis results. IBM Watson is available for public use through IBM’s Bluemix cloud services platform.
IBM’s Watson is listening, watching, talking and understanding with the expanded set of tools, machine learning technologies, and cognitive APIs to make itself more human. Developers can create products, services or applications with more cognitive skills by embedding with IBM Watson to understand how humans interact and react with their applications. IBM Watson has evolved in just 2 years with more than 25 APIs that are powered by approximately 50 technologies. Some of the best services provided by IBM Watson API for building cognitive apps –
- Machine Translation-Helps translate text in different language pairs.
- Message Resonance– To find out the popularity of a phrase or word with a predetermined audience.
- Question and Answers-This service provide direct answers to the queries that are triggered by primary document sources.
- User Modelling– To make predictions about social characteristics of someone from given text.
2) Microsoft Azure Machine Learning API
Azure Machine Learning API helps data scientists publish in minutes which once used to take days after they had developed a feasible model. Azure Machine Learning makes it easy for data scientists to use predictive models in IoT applications by providing APIs for fraud detection, text analytics, recommendation systems and several other business scenarios. The API is built on the machine learning abilities that are available in Microsoft products like Bing and Xbox.
Azure Machine Learning API provides various features like –
- Ability to create a customized configurable R module so that data analysts or data scientists can incorporate their own R language code to train or predict tasks.
- Azure allows data scientists or analysts to include their own Python scripts using various Python libraries for data science like SciPy, SciKit-Learn, NumPy, Pandas, etc. Popular tools like iPython Notebook and various other Python tools for Visual Studio can also be used with the Azure Machine Learning API.
“We have added Python, which is a big favorite of data scientists. There is a huge ecosystem for this. This capability will be powerful for data scientists. We made a lot of improvements and adding Python was part of that. Azure Machine Learning is the platform. You can copy a bit of Python code and plug it into the studio and create an API.”- said Joseph Sirosh, Corporate Vice-President at Microsoft
- Analysts or data scientists can train petabytes of data using one class SVM for anomaly detection or by using PCA or “Learning with Counts”.
- Azure Machine Learning API also supports Spark and Hadoop for big data processing making it an ideal choice irrespective of the platform.
3) Google Prediction API
Google Prediction API lets developers tap into Google’s machine learning algorithms to crunch data and give possible results to make applications smarter. Google Prediction API is a cloud-based machine learning and pattern matching tool for the upsell of opportunity analysis, customer sentiment analysis, churn analysis, spam detection, document classification, purchase prediction, recommendations, intelligent routing and more.
Google Prediction API uses classifiers for programming the API service to make predictions, thus it is enough if the users have some basic programming background without the working knowledge of AI. It reads data from BigQuery and Google Cloud Storage.
Google Prediction API Applications
- Ford uses Google’s Prediction API in its research laboratories to increase the efficiency of driving. It tracks the driver to create a list of places and the routes that the driver visits on daily basis. This helps Ford detect intelligently that if a person is driving a car at the particular time during the weekday then probably it will be a trip to the office. Ford uses Google Prediction API to predict the driver’s behavior on the sensor data captured from their cars.
- California based startup Pondera Solutions that provides Fraud Detection as a Service is built on Google’s Prediction API.
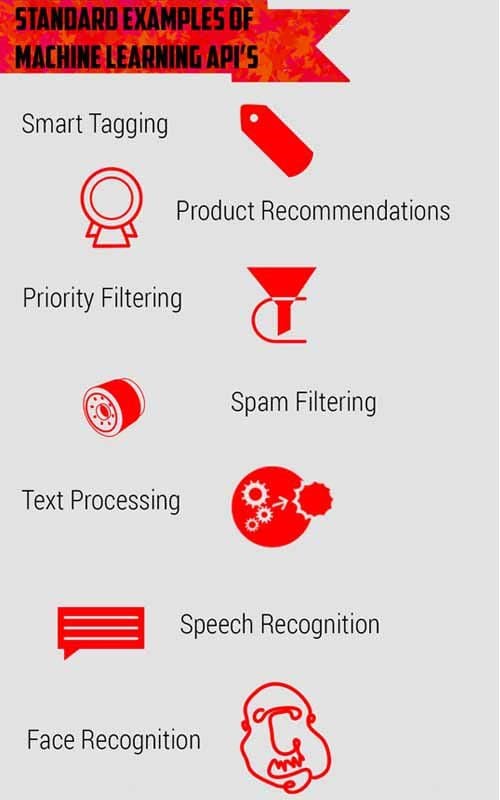
4)Amazon Machine Learning API
Amazon Machine Learning API simplifies the process of making predictions that require lots of expertise around a model building, data cleaning, and statistical analysis.Amazon Machine Learning model deals with only prediction problems and functions by streaming the data past the model and adapting it slowly, depending on the prediction error.Amazon Machine Learning API also provides visualizations based on the prediction performance of the learned model so that the user can gain better insights.
Amazon Machine Learning API although it enforces some kind of UI restrictions or algorithmic restrictions, is user-friendly and wizard-driven, making it a good choice for developers.
Amazon Machine Learning API Use Cases-
- Using AWS API, data scientists or analysts can classify the genre of the song by analyzing the signal level features.
- It can be used for Human Activity Recognition based on an accelerometer, sensor data captured on the smartphone or gyroscope signals to find out if the mobile device is walking upstairs, walking downstairs, lying down vertically or horizontally, sitting still or standing.
- Predict if a user will turn out to be a paid customer by analyzing the user activities during the first week or first month.
- Analyse website activity records to detect fake users, bots or spammers in the system.
All the above use cases have an assumption that there is a need to predict something that is not known at runtime but there is sufficient data and computing power for the Amazon machine learning API to solve the problem at hand.
5) BigML
BigML is user-friendly and developer-friendly Machine learning API that mainly concentrates on decision trees. The motive of BigML is to make predictive analytics easy, understandable and beautiful to the users. It focuses on understanding the business processes and end-user analyst making reports. BigML API provides 3 important modes – Command Line Interface, Web Interface and a RESTful API. The web interface of BigML is pretty cool with features like one-click and gallery.
BigML Use Cases-
- BigML helps create a descriptive model to understand the relationships between the various attributes in the complex data and the predicted attributes so that business analysts can play with the what-if scenarios.
- BigML is useful if the analyst wants to create a predictive model based on past examples. Analysts can build the predictive model using BigML to make a prediction in the batch which can be downloaded as a CSV file for analysis.
- BigML also finds great applications when periodic predictions are to be made. The model can be maintained on the BigML platform and then can be used remotely as and when required using the BigMLer command line interface.
With lots of choices and lots of data, the race is on as to which is the best Machine Learning API- whoever learns fastest is the one who wins. Machine learning algorithms may not be perfect. This should not be a concern as the ultimate decision still rests with the humans. However, these machine learning algorithms cut down the vast number of choices to something that human beings can manage. In future, machine learning will lead to novel innovations that will intensify human capabilities, assist people in making good choices and help navigate through the world in powerful ways.
Very Informative and creative contents. This concept is a good way to enhance knowledge. Thanks for sharing. Continue to share your knowledge through articles like these.
ReplyDeleteData Engineering Services
Data Analytics Services
Machine Learning Services
Data Modernization Services